Lecture 5. Bayesian Graphical Models and Causal Inference
Learning Goals: You should understand the difference between models used for prediction and models describing causal relations between variables. You should be able to describe how wrong conclusions can be drawn from data, if the correct causality structures is not considered. You should know what Directed Acyclic Graphs (DAGs) and structured Causal Models (SCMs) are and how they can be used to describe causality structures. You should know about Chain, Forks and Colliders and what conditional independence they imply. You should also be able to describe Simpson's paradox. You should know the definition and be able to do analysis of conditional independence.
You find the Lecture 5 slides
Download Lecture 5 slides here
You find the Lecture 5 video here:
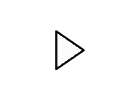
Try out guessthecorrelation.com Links to an external site.
Here is the link to the mentioned additional video on Berkson's paradox Links to an external site.
You will find Additional Material (outside the course) here
Summary of the meeting:
Some code to look at for the lecture session: